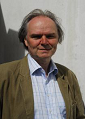
Magnus S Magnusson
University of Iceland, Iceland
Title: Why search for hidden repeated temporal behaviour patterns? T-pattern Analysis (TPA) with Theme
Biography
Biography: Magnus S Magnusson
Abstract
Behavioral analysis has for long been characterized by the use of standard statistical methods developed in other contexts, that is, for quantitative analysis of particular other phenomena or any quantifiable phenomena. Moreover, often due to lack of tools, the counting of behavioural events and states and the measuring of their frequencies and durations has dominated. There has also been some use of multivariate statistics where clouds of points in n-dimensional (data) space reflect relations between behaviours and/or subjects. Hierarchical Cluster Analyses represent such relations in terms of hierarchies of clusters of clusters, however, generally, like with Factor Analysis, the clusters or factors do not describe patterns that recur in time like, for example, repeated words as patterns of letters (phonemes) and repeated phrases as patterns of words or rituals and routines such as greetings and meetings as patterns of simpler behaviours. Standard statistical methods such as sequential analysis are rarely used and typically imply over-simplifying assumptions that may prevent the detection of even abundant repeated patterns. The T-pattern model with its extensions, called the T-system, and corresponding detection algorithms and software, Theme (for Windows), were developed to make such pattern detection feasible and easily available even if computationally intensive. One reason for searching for complex repeated patterns is the possibility of thereby detecting effects of independent variables easily missed by other methods as research increasingly indicates in a number of areas including pharmacology and neuroscience. TPA with Theme allows the analysis of fairly voluminous data, but also of tiny data (just a few events) due to the particular and intensive use of temporal (discrete real-time) information. The T-pattern model with some of its extensions and corresponding algorithms is outlined together with illustrative applications and results.